Mobility Matters Daily #132 - The retail recovery, gentrification and public transport, and the validity of crowdsourced data
With a visualisation on how busy our skies were to boot
Good day friend.
Sometimes your professional life crosses over into things that you do on a voluntary basis. Last night I spent a good proportion of the evening arguing that the Town Council I sit on should offer more than just 2 spaces in its 100+ place car park up for electric vehicle charging points. Thankfully the majority of my fellow councillors were in agreement - with a few exceptions. To the news.
James
The recovery and the impact upon retail travel
Some initial analysis by the Centre for Cities in the UK has indicated that ‘Freedom Day’ had an impact on the number of people shopping in towns and cities across the UK. The recovery in retail spend was most notable in 3 British seaside towns, 2 tourist areas, and 5 other towns and cities. London is notable as one of the worst-recovering areas.
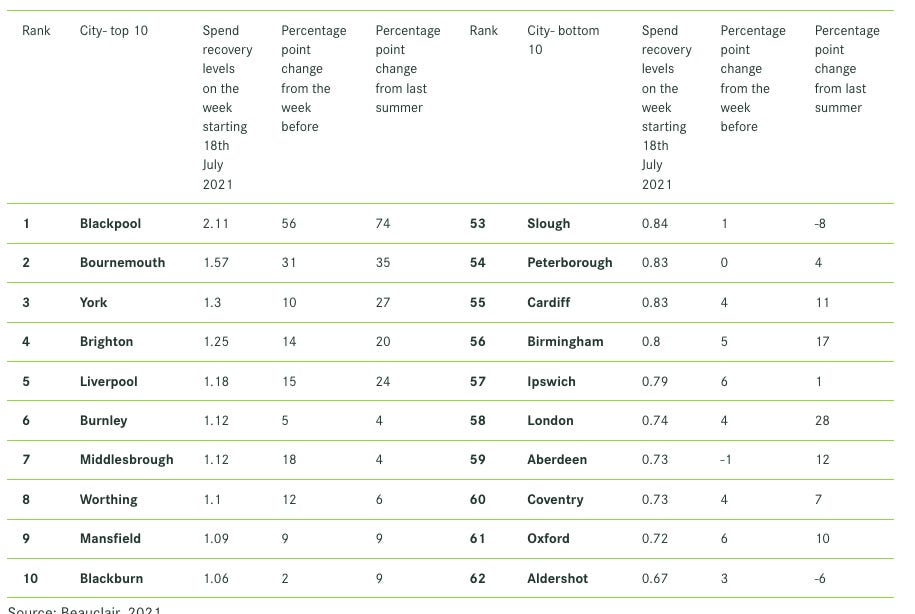
I have a few issues with this analysis - notably its just a percentage comparison and the commentary on tourist areas leading the recovery is a bit misleading (with greatest respect to Blackburn, Mansfield, Middlesborough, and Burnley). But the later analysis comparing worker recovery and retail recovery is interesting.
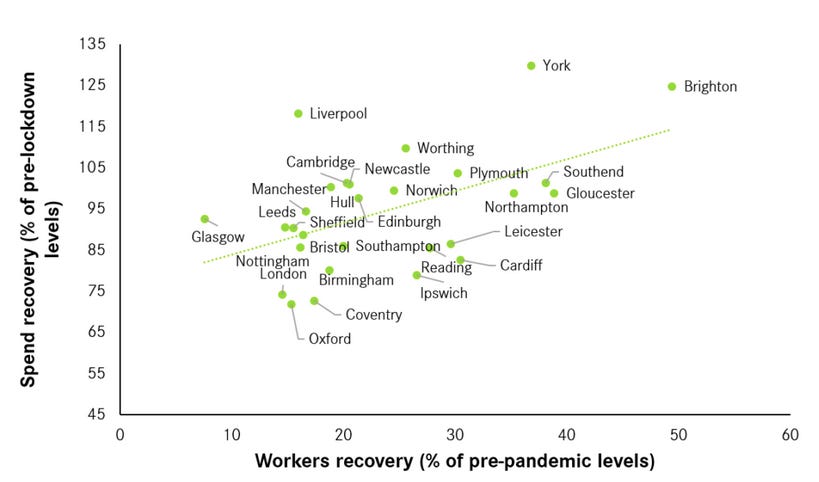
The commentary puts it perfectly:
In these large, economically important cities like London but also Birmingham and Manchester, high street businesses (such as cafes, shops but also evening hospitality venues) rely heavily on office workers, much more than on weekend trade
In the UK, 19% of trips of the average person per year are for shopping (including food). There is a tendancy for commuters to trip chain, not to mention office workers popping out at lunch to get something to eat or have a coffee. If this is not happening, as some very early data is indicating, what does this mean for the retail offer of our towns and cities, and the trips the transport network should cater for?
More research shows the link between gentrification and public transport investment is complicated
A new paper by Malith Fernando, Eva Heinen, and Daniel Johnson explores the role which investing in public transport (in this case the expansion of the Manchester Metrolink) plays in gentrifying areas of a city. Their results indicate that over the longer term, there is evidence of gentrifying characteristics (such as changes in house prices) in areas where there have been improvements in the public transport network, although they stress that transport is but a constituent player in this.
This research adds to a body of work that indicates that when public transport is improved, something happens that could lead to gentrification. But its causes, systems impacts, and scale are unknown, as is transport’s role in stimulating these changes. The feedback loops are complicated, as highlighted by this research paper into the impact of the extension of London’s Jubilee Line and Madrid’s Metrosur. Worthy of more research, to be sure.
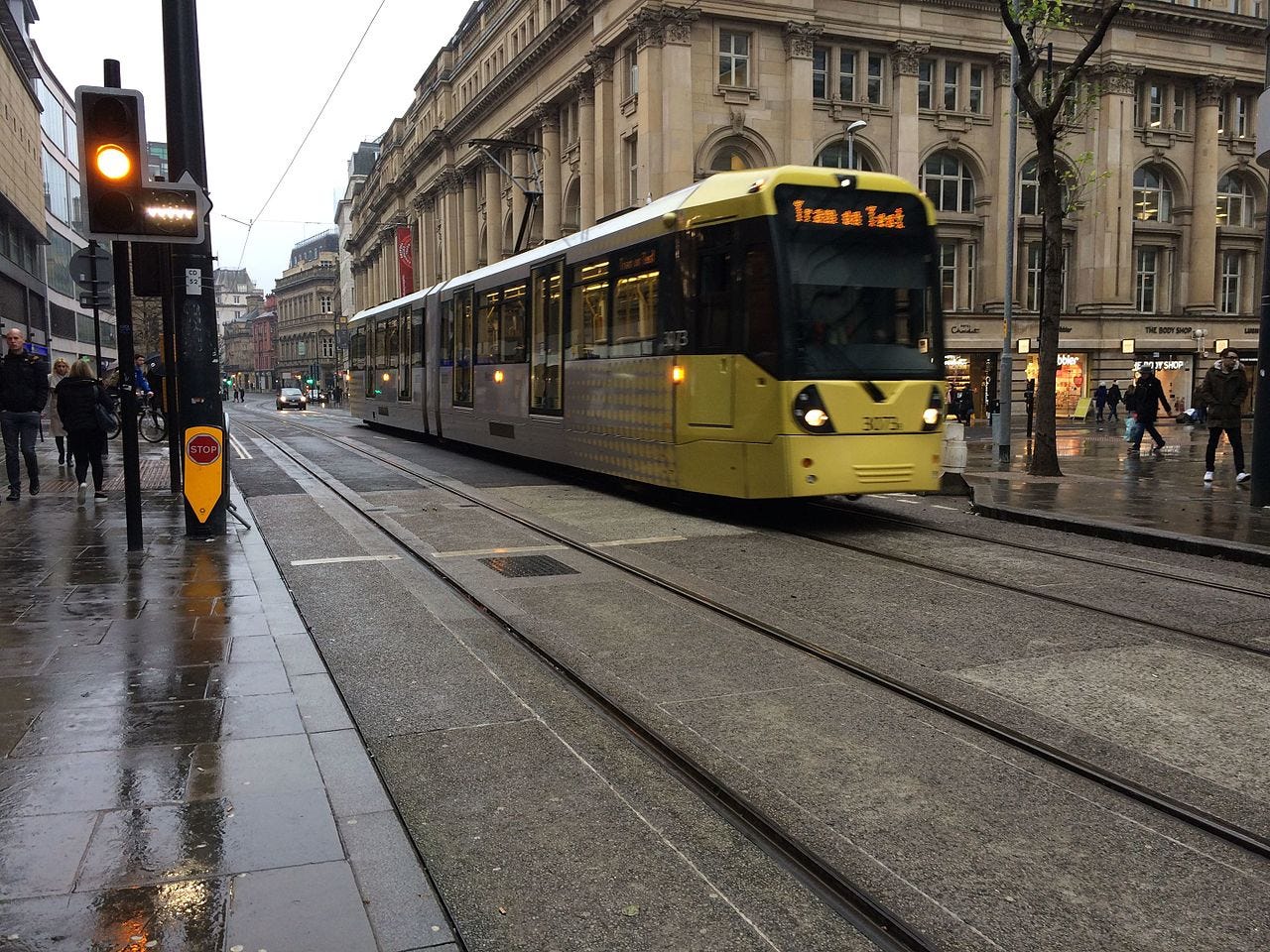
Is crowdsourced data all its cracked up to be?
I liked reading this research paper into how trustworthy crowdsourced data on flooding instances is, specifically from the Waze app in this case. A favourite nugget of information in the summary gave me a wry smile:
Publicly available (but spatially limited) flood incident data from the city (Norfolk, Virginia - my emphasis) in combination with different environmental and topographical factors are used to create a logistic regression model to predict the probability of roadway flooding at any location on the roadway network. The prediction accuracy of the model was found to be 90.5%. When applying this model to crowdsourced Waze flood incident data, 71.7% of the reports were predicted to be trustworthy.
For me, as a general advocate for crowdsourced data (primarily on grounds of the scale of the data giving an indication of change occurring), this gives me a couple of linked thoughts. First is whether a prediction of just over 70% of reports is a good indication of trusworthiness. Second, is whether during a time of flooding when having accurate data in real time to inform potentially life or death decisions, having data where 30% of it is predicted to be inaccurate is any use at all?
To be fair, the paper itself simply reports the results, and does not make a specific recommendation for Waze data to be used in practice in this way, simply stating that the paper…
…demonstrates the potential for using Waze incident report data for roadway flooding detection.
But I want to throw this open to you, who no doubt have more data analysis expertise than I do. What has been your experience with crowdsourced data, and does this paper align with it? Have I misinterpreted the results? Please provide your comments at the end of the newsletter.
Visualisation of the Day
This is a classic one produced by National Air Traffic Services in 2014. This showed how busy the skies were across Europe at the time. The way it the system just kicks in once the sun rises in Europe is insane. Another one is the visualisation of the UK. Watch out for the military jets in this one.
If you do nothing else today, do this
Get your tickets for Mobility Camp. Yeah, I did this one the other day, but you really shouldn’t miss out!